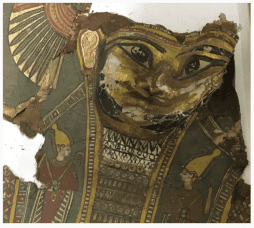
In this thesis, we present the development of deep learning methods for proposing relevant matches of document fragments, applied to the reconstruction of ancient papyri. We have designed a method based on deep Siamese neural networks and a self-supervised approach to overcome the problem of the small amount of annotated training data available in this domain.
This work is part of the GESHAEM project, a scientific research initiative funded by the European Research Council (ERC). Its objective is to study a papyrological corpus, the Jouguet collection of the Sorbonne, composed of papyri that were torn to make funerary ornaments. These papyri are for the most part administrative and fiscal documents whose content is very useful for historians in order to better understand the way these ancient societies were organized. However, due to the fact that the papyri were torn up about 2000 years ago, and that they have been very degraded due to time and conservation conditions, their study requires a great deal of reconstruction work by papyrologists. This is a long and tedious process if done by hand, as the collection is composed of hundreds of fragments.
The objective of this work is thus to compute fragment matching suggestions to papyrologists in order to speed up the reconstruction process. We propose a patch-based approach using deep Siamese neural networks to compute a similarity score between two papyrus fragments. The difficulty of obtaining large amounts of annotated data makes it difficult to build a model that works on different types of documents. For this purpose, we propose a self-supervised learning methodology exploiting the intrinsic structure of documents. This method allows to train deep Siamese neural networks without the need of annotated data. We have also built a ready-to-use database based on the University of Michigan papyrus collection, which we have opened to the community.
Jury members:
- Hardold Mouchère Prof. University of Nantes Prof. INSA Lyon
- Véronique Eglin Prof. INSA Lyon
- Andreas Fischer Prof. HES-SO
- Marie-Pierre Chaufray CR CNRS, Ausonius
- Daniel Stoekl Ben Ezra DE EPHE
- Marie Beurton-Aimar MCF-HDR University of Bordeaux
- Nicholas Journet MCF-HDR University of Bordeaux
Link(s) : https://u-bordeaux-fr.zoom.us/j/87828836907